Unlocking the Power of an Annotation Tool for Image Segmentation
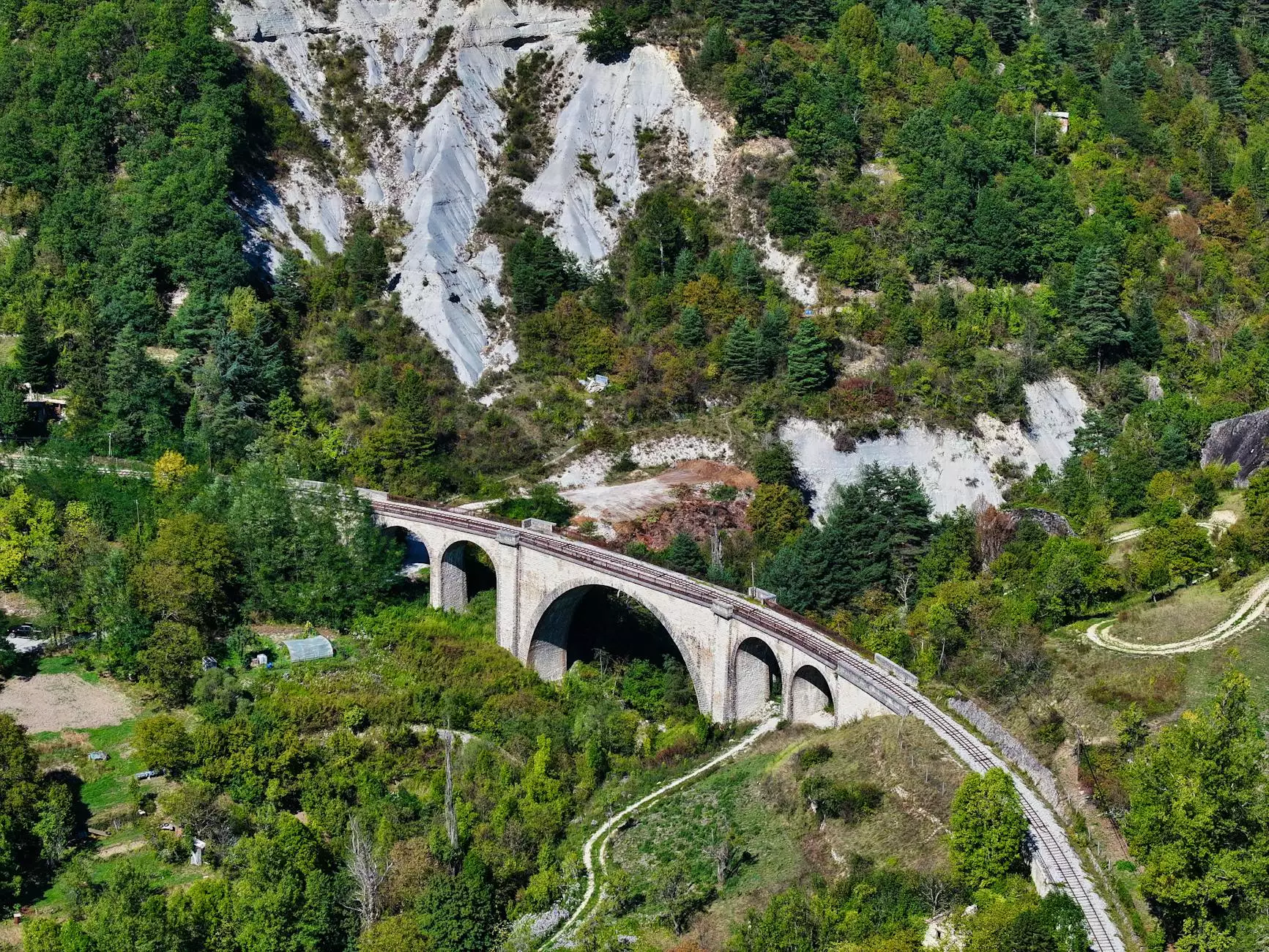
In today's data-driven world, the ability to accurately analyze and interpret visual information is more pivotal than ever. One of the essential components in the realm of machine learning and artificial intelligence is the effective segmentation of images. This is where an annotation tool for image segmentation comes into play, serving as a crucial asset for software developers and data scientists alike.
Understanding Image Segmentation
Image segmentation is the process of partitioning an image into multiple segments or regions, making it easier to analyze and understand. By isolating various parts of an image, developers can train their algorithms to recognize patterns, identify objects, and make predictions based on the visual data.
For example, in the field of autonomous driving, an image segmentation tool can differentiate between pedestrians, vehicles, and road signs, allowing the system to react accordingly. This is just one of the many applications where accurate segmentation is vital.
Why Use an Annotation Tool?
Utilizing an annotation tool for image segmentation offers numerous advantages that can significantly enhance your workflow and project outcomes:
- Efficiency: Automated tools speed up the annotation process, allowing you to label images faster than manual methods.
- Accuracy: High-quality annotation tools come with features that help to reduce human error, ensuring that the segmented images are as precise as possible.
- Scalability: As your project scales, an effective tool can handle larger datasets without compromising on performance.
- Collaboration: Many annotation tools enable multiple users to work simultaneously, fostering better collaboration among team members.
Key Features of a Quality Annotation Tool
When selecting an annotation tool for image segmentation, it's critical to consider the features that will best serve your project's needs:
1. User-Friendly Interface
A clean, intuitive interface can dramatically improve productivity. Look for tools that offer drag-and-drop functionality and easy navigation for annotators.
2. Variety of Annotation Types
Choose a tool that supports various annotation types, including bounding boxes, polygons, and semantic segmentation. This flexibility allows you to adapt the tool for different projects and requirements.
3. Integration Capabilities
Ensure that the annotation tool can easily integrate with your existing software development ecosystem, including APIs, machine learning frameworks, and data storage solutions.
4. Quality Control Features
Incorporate tools that facilitate quality control, such as review processes and feedback loops. This ensures that annotations meet the desired standards before being used in training models.
Best Practices for Using an Annotation Tool
To maximize the effectiveness of an annotation tool for image segmentation, consider the following best practices:
1. Define Clear Guidelines
Provide annotators with detailed guidelines on the segmentation task to avoid discrepancies and enhance consistency across annotations. This is especially crucial in projects involving multiple annotators.
2. Regular Training Sessions
Conduct training sessions to familiarize annotators with the tool's features and functionalities. Continuous education ensures that your team is proficient and up-to-date with the latest practices.
3. Use Pre-Annotation When Possible
Some tools offer pre-annotation features, using existing models to provide initial guesses. This can save time and reduce the manual workload, though human oversight is still necessary.
4. Perform Regular Quality Checks
Investing time in regular quality checks can help maintain high standards. Running quality assurance audits ensures that the segmentation accurately represents the intended objects or areas.
The Impact of Image Segmentation on AI Development
The relationship between annotation tools and the advancement of artificial intelligence (AI) cannot be overstated. Image segmentation directly influences the performance of AI models, particularly in fields such as:
- Healthcare: For diagnosing conditions using medical imaging.
- Automotive: Essential for the development of self-driving cars.
- Security: Video surveillance systems rely heavily on accurate object recognition.
- Retail: Enhancing customer experiences through personalized image and video content.
Real-World Applications of Annotation Tools
Annotated data sets generated through image segmentation serve as the backbone for numerous applications across various industries:
1. Autonomous Vehicles
In the automotive industry, self-driving technology depends on precise segmentation of the surroundings. Vehicles must identify roads, obstacles, pedestrians, and potential hazards in real-time. An annotation tool for image segmentation enables the creation of robust datasets to train these systems effectively.
2. Medical Imaging
In healthcare, accurate segmentation of medical images allows for better diagnosis and treatment planning. For instance, segmenting tumors in MRI images can significantly improve the accuracy of radiotherapy treatments, demonstrating the critical nature of effective annotation tools in this domain.
3. Agricultural Technology
In agriculture, image segmentation can be applied to closely monitor crop health and optimize yield. Using drones equipped with advanced imaging technology, farmers can segment various plant species, detect pests, and manage resources more efficiently.
4. Retail and E-Commerce
For retailers, the ability to segment images allows for better visual merchandising. Automated systems can categorize products based on detailed attributes, which enhances searchability and improves customer experiences.
The Future of Annotation Tools in Image Segmentation
As technology continues to evolve, so too do the tools available for image segmentation. The future of annotation tools is bright and promising with advancements in:
1. Machine Learning Integration
Tools that incorporate machine learning algorithms can not only improve automatic segmentation but also learn from user input, becoming increasingly accurate over time.
2. Augmented Reality and Virtual Reality
With the rise of AR and VR, annotation tools will need to adapt to new mediums, providing capabilities that allow for real-time segmentation in three-dimensional spaces.
3. Enhanced User Experiences
Future tools will focus on user experience, improving interfaces and employing advanced visual analytics to help users gain insights quickly.
Conclusion
The significance of using a reliable annotation tool for image segmentation cannot be overstated in today’s technological landscape. As the demand for high-quality visual data continues to rise, so will the need for advanced tools capable of delivering precision and efficiency. By leveraging these tools effectively, software developers and data scientists can push the boundaries of innovation across a multitude of industries, paving the way for groundbreaking advancements in artificial intelligence, machine learning, and beyond.
Investing in an annotation tool for image segmentation not only enhances the accuracy of your data but also streamlines your processes, driving your projects toward successful outcomes more efficiently than ever.